2 Introduction - The Choice that Doesn’t Matter
The very first (and intimidating) choice a novice hacker faces is which is programming language to learn. Unfortunately the medium popularily summed up as the internet offers a lot of really really good advice on the matter. The problem is, however, that this advice does not necessarily agree which language is the best for research. In the realm of data science – get accustomed to that label if you are a scientist who works with data – the debate basically comes down to two languages: The R Language for Statistical Computing and Python.
At least to me, there is only one valid advice: It simply does NOT matter. If you stick around in data science long enough you will eventually get in touch with both languages and in turn learn both. There is a huge overlap of what you can do either of those languages. R came out of the rather specific domain of statiscs 25+ years ago and made its way to a more general programming language thanks to 15K+ extension packages (and counting). Built by a mathmatician, Python continues to be as general purpose as it’s ever been. But it got more scientific, thanks to extension packages of its own such as pandas, SciPy or numPy. As a result there is a huge overlap of what both languages can do and both will extend your horizon in unprecendented fashion if you did not use a full fledged programming language for your analysis before.
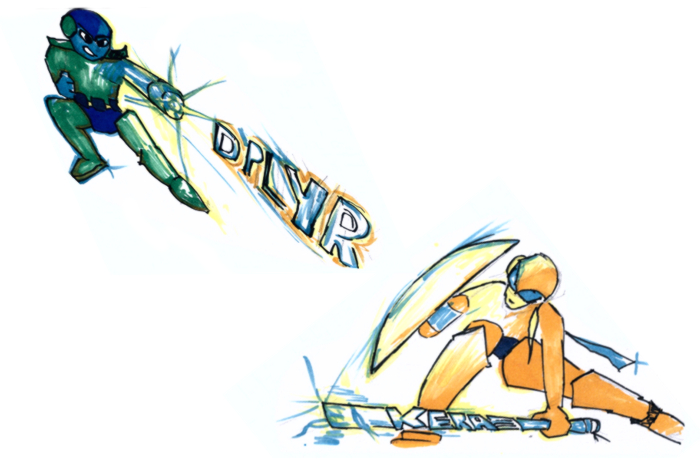
But why is there such a heartfelt debate online, if it doesn’t matter? Let’s pick up a random argument from this debate: R is easier to set up and Python is better for machine learning. If you worked with Java or another environment that’s rather tricky to get going, you are hardened and might not cherish easy onboarding. If you got frustrated before you really started, you might feel otherwise. You may just have been unlucky making guesses about a not so well documented paragraph, trying to reproduce a nifty machine learning blog post. Just because you installed the wrong version of Python or didn’t manage to make sense of virtualenv right from the beginning.
The point is, rest assured, if you just start doing analytics using a programming languages both languages are guaranteed to carry you a long way. There is no way to tell for sure which one will be the more dominant language in 10 years from now or whether both still be around holding their ground the way they do now. But once you reached a decent software carpentry level in either language, it will help you a lot learning the other. If your peers work with R, start with R, if your close community works with Python, start with Python. If you are in for the longer run either language will help you understand the concepts and ideas of programming with data. Trust me, there will be a natural opportunity to get to know the other.
2.2 How to Read this Book?
Hacking for Social Sciences is written based on the experience of helping students and seasoned researchers of different fields with their data management, processing and communication of results. A part of the book contains the information I wish I had when I started a PhD in economics. Part of the book is written years after said PhD was completed and with the hindsight of 10+ years in academia. Every page of the book is written with the belief that the future is OPEN and it is up to our generation of researchers to shape it.
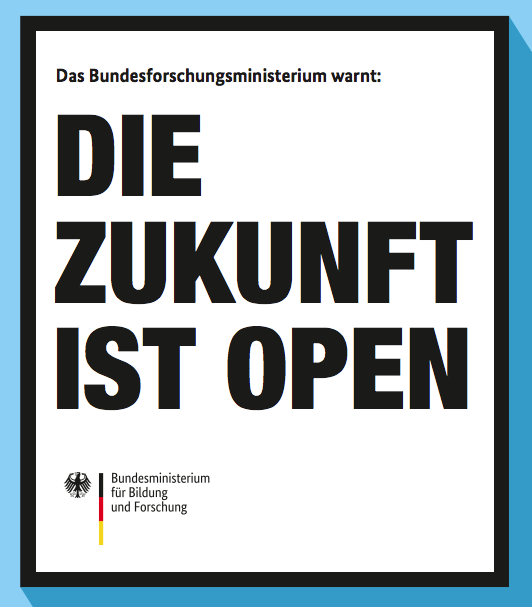
If you came to
🌸
pick, you’re welcome, too (but a bit early to the party though). This book will grow along the 2020 course ‘Hacking for Social Science’ and hopefully be finished in its first version by the end of the semester / year. Next up are chapters on the Big Picture of Open Source Software for hacking data and Git version control.